Decentralized Federated Learning: A New Era in Artificial Intelligence 🧠
Posted September 15, 2023 by Enrique Tomás Martínez Beltrán ‐ 2 min read
Funded by: Fundación Séneca (Science and Technology Agency of the Region of Murcia)
Grant: 21629/FPI/21
In an era where Artificial Intelligence (AI) plays a pivotal role, Decentralized Federated Learning (DFL)1 emerges as a beacon of hope, particularly in scenarios demanding utmost privacy and efficiency. It is becoming a cornerstone in facilitating collaborative learning across diverse devices while ensuring data remains local. While the concept of Federated Learning is not new, the shift from a centralized model to a decentralized approach significantly amplifies its potential.
DFL is a federated system in which communications are decentralized among the participants of a network. Therefore, it brings a new wave of opportunities, including mitigating the risk of a single point of failure, reducing communication costs, enabling a truly scalable solution, and enhancing trust between participants by minimizing reliance on a central authority.
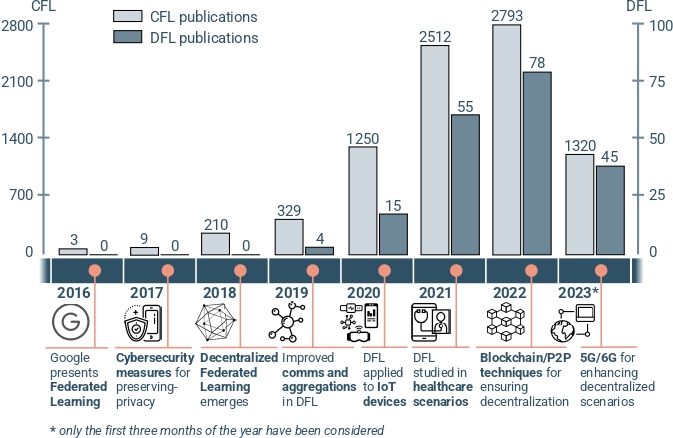
The integration of DFL introduces its unique challenges that require detailed exploration and comprehension. The transformative realm of DFL is vast, encompassing a wide range of intricacies. At its core lies the importance of privacy-preserving machine learning. By examining the motivations and objectives behind DFL, we can better understand its distinctions from centralized approaches. Through an in-depth look at i) DFL architectures, ii) components, iii) topologies, iv) communication protocols, and v) security methodologies, the foundational mechanisms become clear.

Additionally, pivotal aspects of trust management are addressed, and essential optimization strategies, including algorithm selections and performance evaluations, are highlighted. Shifting the focus to the tangible impacts, real-world applications of DFL in sectors like healthcare, manufacturing, mobile services, military, and vehicles come to the fore. Such implementations showcase DFL’s role in tackling present-day issues, underscoring its practical significance and revolutionary potential.
Another application scenario is in cyberattack detection. As cyberattacks grow more frequent and sophisticated, detection becomes increasingly challenging. DFL could empower a network of computers to train an ML model to identify suspicious behavior patterns entirely privately and decentralized. This setup could prevent attackers from accessing user data and speed up the detection of such cyber threats. Technologies like Blockchain support DFL, ensuring the immutability of contributions from each network participant and providing a solid foundation for data traceability or identifying dishonest participants.
Martinez Beltrán, E. T., Quiles Pérez, M., Sánchez Sánchez, P., López Bernal, S., Bovet, G., Gil Pérez, M., Martínez Pérez, G., & Huertas Celdrán, A. (2023). Decentralized Federated Learning: Fundamentals, State of the Art, Frameworks, Trends, and Challenges. IEEE Communications Surveys & Tutorials doi: 10.1109/COMST.2023.3315746 ↩︎